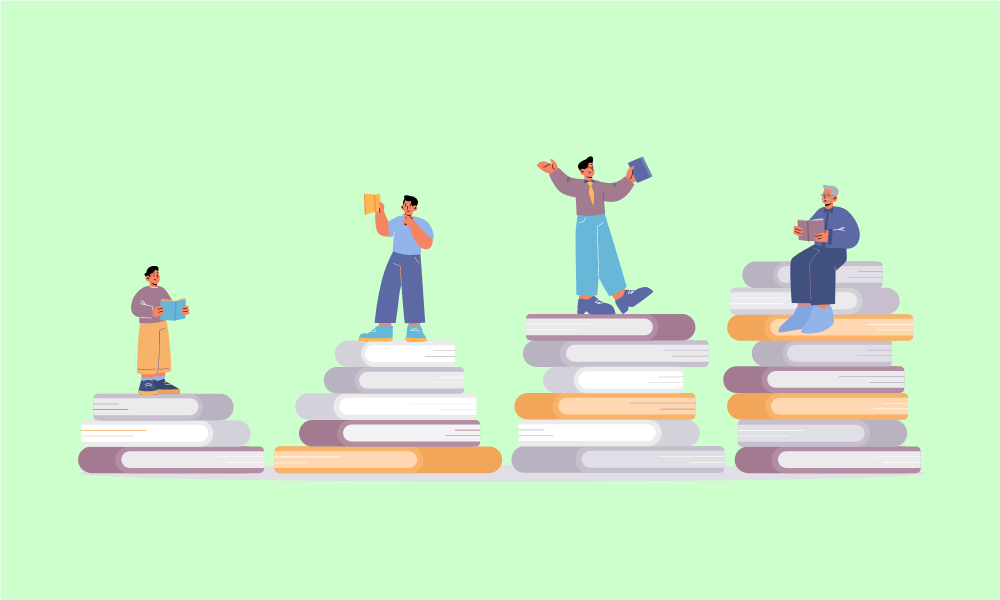
Publications
Here is a list of my publications, including publications I co-authored. By clicking on the publication tile, you will be redirected to the abstract and further informations.
Design Principles for Falsifiable, Replicable, Reproducible Empiricial Machine Learning Research
D. Vranjes, J. Ehrhardt, R. Heesch, L. Moddemann, H. Steude, O. Niggemann, “Design Principles for Falsifiable, Replicable, Reproducible Empiricial Machine Learning Research,” *Conference on the Principles of Diagnosis - DX, 2024*. doi:[https://doi.org/10.4230/OASIcs.DX.2024.7](https://doi.org/10.4230/OASIcs.DX.2024.7).
A lazy Approach to Neural Numerical Planning with Control Parameters
R. Heesch, A. Cimatti, J. Ehrhardt, A. Diedrich, O. Niggemann, “A lazy Approach to Neural Numerical Planning with Control Parameters,” *ECAI - European Conference on Artificial Intelligence, 2024*. doi:[10.3233/FAIA241000](https://doi.org/10.3233/FAIA241000).
Using Modular Neural Networks for Anomaly Detection in Cyber-Physical Systems
J. Ehrhardt, P. Overlöper, D. Vranjes, H. Steude, A. Diedrich, O. Niggemann, “Using Modular Neural Networks for Anomaly Detection in Cyber-Physical Systems,” *ETFA - IEEE Conference on Emerging Technologies and Factory Automation, 2024*. doi:[10.1109/ETFA61755.2024.10711115](https://doi.org/10.1109/ETFA61755.2024.10711115).
Diagnosis Driven Anomaly Detection for Cyber-Physical Systems
H. S. Steude, L. Moddemann, A. Diedrich, J. Ehrhardt, and O. Niggemann, “Diagnosis driven Anomaly Detection for CPS.” arXiv, 2023. doi: 10.48550/ARXIV.2311.15924. doi:[10.48550/ARXIV.2311.15924](10.48550/ARXIV.2311.15924).
Using FliPSi to Generate Data for Machine Learning Algorithms
A. Liebert, C. Wittke, J. Ehrhardt, R. Jaufmann, N. Widulle, S. Eilermann, M. Krantz, O. Niggemann, “Using FliPSi to Generate Data for Machine Learning Algorithms,” *ETFA - IEEE Conference on Emerging Technologies and Factory Automation, 2023*. doi:[10.1109/ETFA54631.2023.10275500](http://dx.doi.org/10.1109/ETFA54631.2023.10275500).
Learning Process Steps as Dynamical Systems for a Sub-Symbolic Approach of Process Planning in Cyber-Physical Production Systems
Ehrhardt, J., Heesch, R., Niggemann, O. (2024). Learning Process Steps as Dynamical Systems for a Sub-Symbolic Approach of Process Planning in Cyber-Physical Production Systems. In: *Nowaczyk, S., et al. Artificial Intelligence. ECAI 2023 International Workshops. ECAI 2023. Communications in Computer and Information Science, vol 1948. Springer, Cham*. [10.1007/978-3-031-50485-3_34](https://doi.org/10.1007/978-3-031-50485-3_34).
Integrating Machine Learning into an SMT-based Planning Approach for Production Planning in Cyber-Physical Production Systems
Heesch, R., Ehrhardt, J., Niggemann, O. (2024). Integrating Machine Learning into an SMT-Based Planning Approach for Production Planning in Cyber-Physical Production Systems. In: *Nowaczyk, S., et al. Artificial Intelligence. ECAI 2023 International Workshops. ECAI 2023. Communications in Computer and Information Science, vol 1948. Springer, Cham*. doi: [10.1007/978-3-031-50485-3_33](https://doi.org/10.1007/978-3-031-50485-3_33).
FliPSi: Generating Data for the Training of Machine Learning Algorithms for CPPS
M. Krantz et al., “FliPSi: Generating Data for the Training of Machine Learning Algorithms for CPPS,” *Annual Conference of the PHM Society, vol. 14, no. 1. PHM Society, Oct. 28, 2022*. doi: [10.36001/phmconf.2022.v14i1.3229](https://doi.org/10.36001/phmconf.2022.v14i1.3229).
An AI benchmark for Diagnosis, Reconfiguration & Planning
J. Ehrhardt, M. Ramonat, R. Heesch, K. Balzereit, A. Diedrich, and O. Niggemann, “An AI benchmark for Diagnosis, Reconfiguration & Planning,” *2022 IEEE 27th International Conference on Emerging Technologies and Factory Automation (ETFA). IEEE, Sep. 06, 2022*. doi: [10.1109/etfa52439.2022.9921546](https://doi.org/10.1109/ETFA52439.2022.9921546).
Creating Automatic Semantic Device Descriptions for Brownfield Industrial Robots
J. Ehrhardt, A. Nordhausen, Alexander Guhl, Marcel Lewke, Constantin Hildebrandt, Oliver Niggemann, “Creating Automatic Semantic Device Descriptions for Brownfield Industrial Robots,” *SAMPE Europe Conference*.
A Machine Learning Approach for Revenue Management in Cloud Manufacturing
V. Adomat, J. Ehrhardt, C. Kober, M. Ahanpanjeh, and J. P. Wulfsberg, “A Machine Learning Approach for Revenue Management in Cloud Manufacturing,” *Procedia CIRP, vol. 118. Elsevier BV, pp. 342–347, 2023*. doi: [10.1016/j.procir.2023.06.059](https://doi.org/10.1016/j.procir.2023.06.059).
Omission of Information: Identifying Political Slant via an Analysis of Co-occurring Entities
J. Ehrhardt, T. Spinde, A. Vardasbi, and F. Hamborg, “Omission of Information: Identifying Political Slant via an Analysis of Co-occurring Entities,” *Universität Regensburg, 2021*. doi: [10.5283/EPUB.44939](https://epub.uni-regensburg.de/id/eprint/44939).
The Digital Shadow: Developing a Universal Model for the Automated Optimization of Cyber-Physical Production Systems Based on Real-time Data
J. M. Ehrhardt and C. T. Hoffmann, “The Digital Shadow: Developing a universal model for the automated optimization of cyber-physical production systems based on real-time data,” *Procedia CIRP, vol. 93. Elsevier BV, pp. 304–310, 2020*. doi: [10.1016/j.procir.2020.03.069](https://doi.org/10.1016/j.procir.2020.03.069).